Table of contents
Subscribe to DVS 2024
Sign up now to get access to the library of members-only issues.
Building or Buying: Strategies for Scaling AI Adoption in Finance and Enterprise
Building or Buying: Strategies for Scaling AI Adoption in Finance and Enterprise
Building or Buying: Strategies for Scaling AI Adoption in Finance and Enterprise
Insights & Trends
Insights & Trends
7 minutes
7 minutes
Oct 23, 2024
Oct 23, 2024
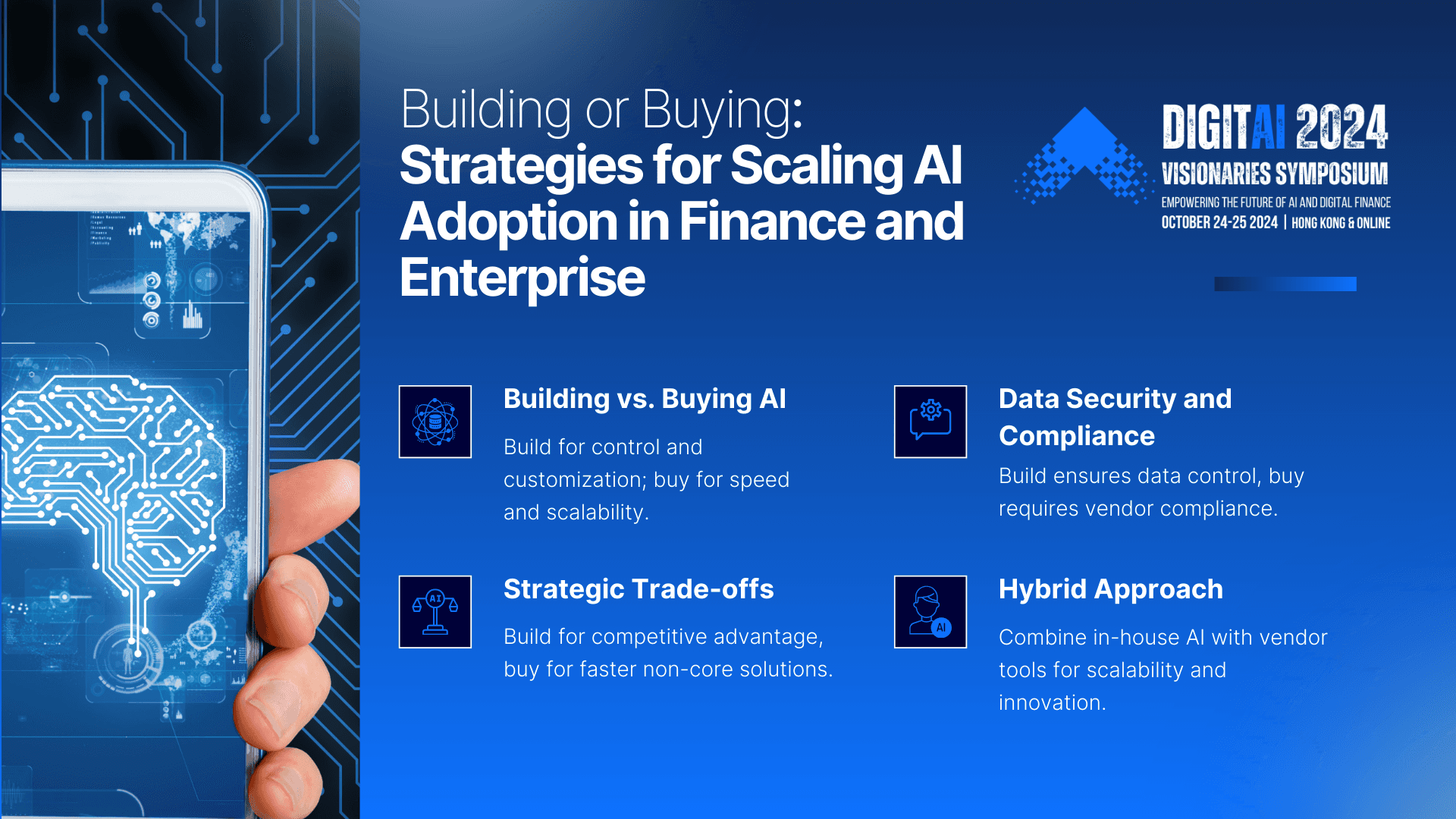
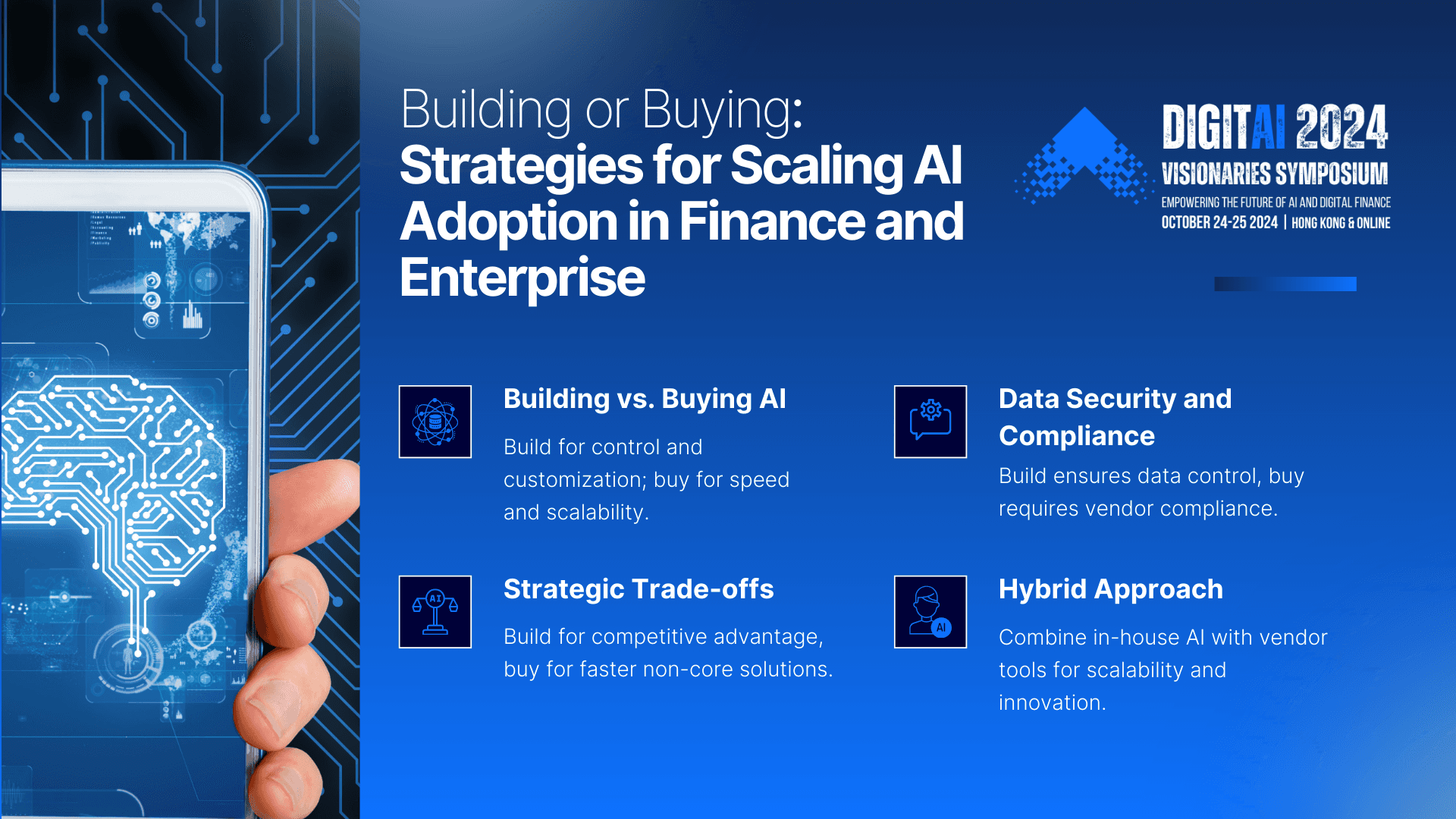
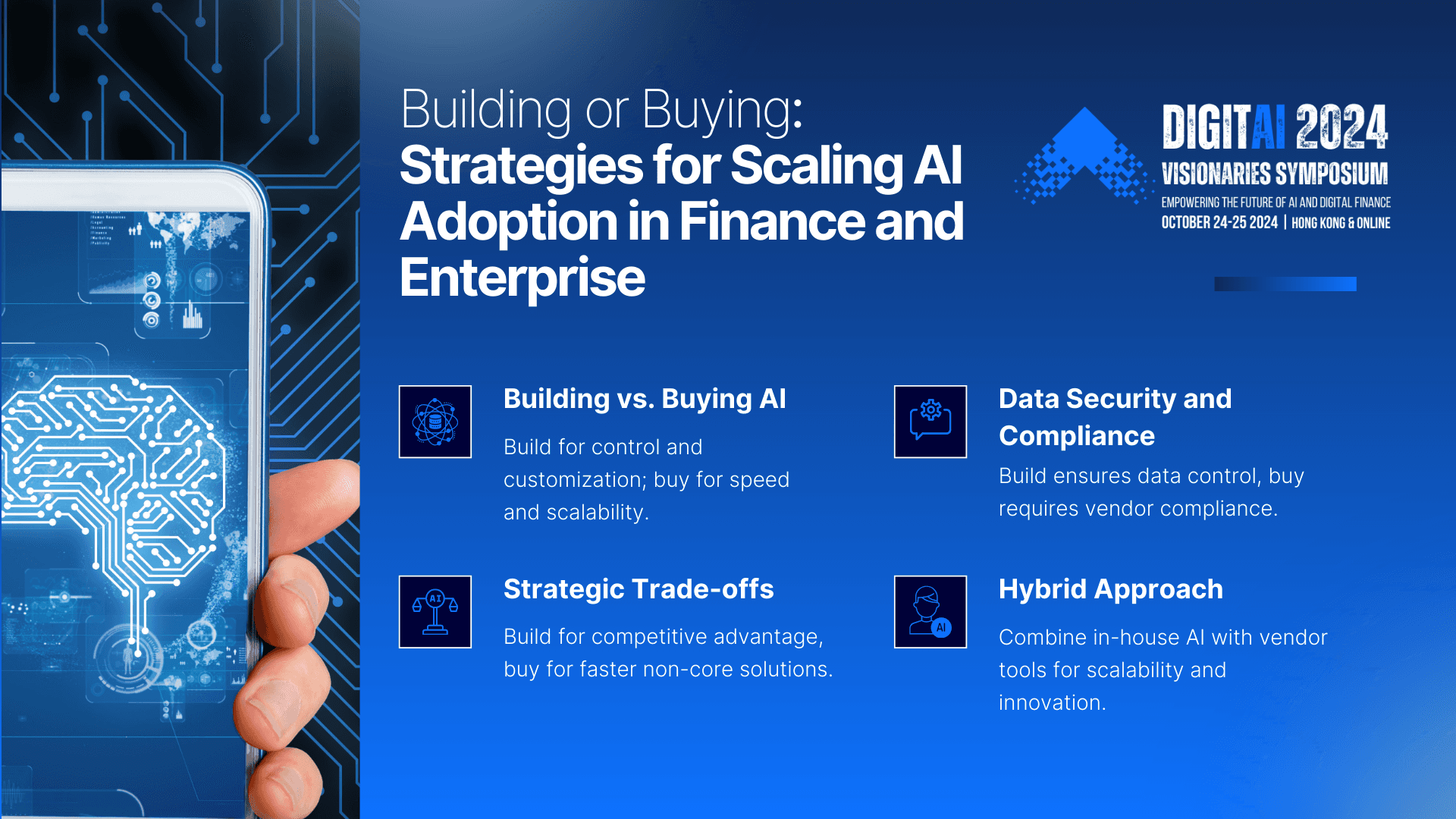
Building AI solutions in-house offers greater control over data management and compliance with stringent regulations like GDPR. However, buying AI from vendors requires careful oversight to ensure data security and compliance, especially in highly regulated industries like finance.
Building proprietary AI systems allows for deep customization and competitive differentiation, while buying AI solutions provides faster scalability. A hybrid approach can offer the best balance, leveraging both in-house innovation and vendor capabilities for non-core functions.
Hear from industry leaders at the Digital Visionaries Symposium 2024. Don’t miss this panel discussion – “Building or Buying: Strategies for Scaling AI Adoption in Finance and Enterprise” – on October 24, 2024. Participate in person or online for actionable insights into AI and digital finance.
Building AI solutions in-house offers greater control over data management and compliance with stringent regulations like GDPR. However, buying AI from vendors requires careful oversight to ensure data security and compliance, especially in highly regulated industries like finance.
Building proprietary AI systems allows for deep customization and competitive differentiation, while buying AI solutions provides faster scalability. A hybrid approach can offer the best balance, leveraging both in-house innovation and vendor capabilities for non-core functions.
Hear from industry leaders at the Digital Visionaries Symposium 2024. Don’t miss this panel discussion – “Building or Buying: Strategies for Scaling AI Adoption in Finance and Enterprise” – on October 24, 2024. Participate in person or online for actionable insights into AI and digital finance.
Building AI solutions in-house offers greater control over data management and compliance with stringent regulations like GDPR. However, buying AI from vendors requires careful oversight to ensure data security and compliance, especially in highly regulated industries like finance.
Building proprietary AI systems allows for deep customization and competitive differentiation, while buying AI solutions provides faster scalability. A hybrid approach can offer the best balance, leveraging both in-house innovation and vendor capabilities for non-core functions.
Hear from industry leaders at the Digital Visionaries Symposium 2024. Don’t miss this panel discussion – “Building or Buying: Strategies for Scaling AI Adoption in Finance and Enterprise” – on October 24, 2024. Participate in person or online for actionable insights into AI and digital finance.
The integration of artificial intelligence (AI) in finance and enterprise is no longer a question of "if" but "how." As companies strive to maintain a competitive edge, they must decide whether to build proprietary AI systems or buy pre-built AI solutions from external vendors.
This insight explores the strategic factors behind this decision and highlights the impact on scalability, compliance, and long-term growth through real-world examples.
The integration of artificial intelligence (AI) in finance and enterprise is no longer a question of "if" but "how." As companies strive to maintain a competitive edge, they must decide whether to build proprietary AI systems or buy pre-built AI solutions from external vendors.
This insight explores the strategic factors behind this decision and highlights the impact on scalability, compliance, and long-term growth through real-world examples.
The integration of artificial intelligence (AI) in finance and enterprise is no longer a question of "if" but "how." As companies strive to maintain a competitive edge, they must decide whether to build proprietary AI systems or buy pre-built AI solutions from external vendors.
This insight explores the strategic factors behind this decision and highlights the impact on scalability, compliance, and long-term growth through real-world examples.
Evolving Market Dynamics and Regulatory Pressures
Evolving Market Dynamics and Regulatory Pressures
Evolving Market Dynamics and Regulatory Pressures
Finance and enterprise companies operate in a landscape shaped by increasing regulatory pressures and market dynamics. For instance, the EU’s General Data Protection Regulation (GDPR) has had a significant influence on how companies handle personal data, leading firms to reconsider how AI systems are developed and managed. In the U.S., financial institutions are regulated by strict mandates under the Dodd-Frank Act, which governs transparency and accountability.
A notable example of a company navigating these regulatory pressures is HSBC, one of the world’s largest banking institutions. To address regulatory concerns, HSBC chose to build a proprietary AI platform for fraud detection. The system integrates advanced machine learning models trained on HSBC’s internal datasets, allowing the bank to comply with complex international regulations while maintaining control over its data management.
In contrast, companies like Capital One opted to partner with Amazon Web Services (AWS) to buy and integrate AI-driven cloud solutions. This approach enabled them to scale AI faster and comply with evolving regulatory requirements while leveraging AWS’s compliance certifications
Finance and enterprise companies operate in a landscape shaped by increasing regulatory pressures and market dynamics. For instance, the EU’s General Data Protection Regulation (GDPR) has had a significant influence on how companies handle personal data, leading firms to reconsider how AI systems are developed and managed. In the U.S., financial institutions are regulated by strict mandates under the Dodd-Frank Act, which governs transparency and accountability.
A notable example of a company navigating these regulatory pressures is HSBC, one of the world’s largest banking institutions. To address regulatory concerns, HSBC chose to build a proprietary AI platform for fraud detection. The system integrates advanced machine learning models trained on HSBC’s internal datasets, allowing the bank to comply with complex international regulations while maintaining control over its data management.
In contrast, companies like Capital One opted to partner with Amazon Web Services (AWS) to buy and integrate AI-driven cloud solutions. This approach enabled them to scale AI faster and comply with evolving regulatory requirements while leveraging AWS’s compliance certifications
Finance and enterprise companies operate in a landscape shaped by increasing regulatory pressures and market dynamics. For instance, the EU’s General Data Protection Regulation (GDPR) has had a significant influence on how companies handle personal data, leading firms to reconsider how AI systems are developed and managed. In the U.S., financial institutions are regulated by strict mandates under the Dodd-Frank Act, which governs transparency and accountability.
A notable example of a company navigating these regulatory pressures is HSBC, one of the world’s largest banking institutions. To address regulatory concerns, HSBC chose to build a proprietary AI platform for fraud detection. The system integrates advanced machine learning models trained on HSBC’s internal datasets, allowing the bank to comply with complex international regulations while maintaining control over its data management.
In contrast, companies like Capital One opted to partner with Amazon Web Services (AWS) to buy and integrate AI-driven cloud solutions. This approach enabled them to scale AI faster and comply with evolving regulatory requirements while leveraging AWS’s compliance certifications
Strategic Trade-Offs: Building vs. Buying AI
Strategic Trade-Offs: Building vs. Buying AI
Strategic Trade-Offs: Building vs. Buying AI
The strategic decision to build or buy AI solutions often hinges on time-to-market and the ability to maintain competitive differentiation. Building proprietary AI systems allows companies to tailor solutions that align with specific business needs.
For instance, JPMorgan Chase has built an internal AI solution, COiN (Contract Intelligence), which analyzes commercial contracts to identify risks. By developing this in-house, JPMorgan Chase ensures that the AI system integrates seamlessly with its existing risk management processes and gives them a long-term competitive advantage.
On the flip side, companies like Goldman Sachs have chosen a hybrid approach. While building proprietary AI for their core investment functions, they rely on third-party AI vendors for tasks like HR and customer support. This mix of buying and building helps Goldman Sachs accelerate AI implementation in non-core areas while focusing its internal resources on high-impact AI innovations.
For smaller enterprises or those looking for faster AI integration, buying pre-built AI solutions offers a quick and cost-effective alternative. For example, Zest AI, an AI-powered lending platform, enables financial institutions to quickly adopt AI without investing in building models from scratch. The plug-and-play nature of Zest AI's platform allows firms to tap into AI for loan underwriting and credit risk assessments, significantly shortening the time-to-market
The strategic decision to build or buy AI solutions often hinges on time-to-market and the ability to maintain competitive differentiation. Building proprietary AI systems allows companies to tailor solutions that align with specific business needs.
For instance, JPMorgan Chase has built an internal AI solution, COiN (Contract Intelligence), which analyzes commercial contracts to identify risks. By developing this in-house, JPMorgan Chase ensures that the AI system integrates seamlessly with its existing risk management processes and gives them a long-term competitive advantage.
On the flip side, companies like Goldman Sachs have chosen a hybrid approach. While building proprietary AI for their core investment functions, they rely on third-party AI vendors for tasks like HR and customer support. This mix of buying and building helps Goldman Sachs accelerate AI implementation in non-core areas while focusing its internal resources on high-impact AI innovations.
For smaller enterprises or those looking for faster AI integration, buying pre-built AI solutions offers a quick and cost-effective alternative. For example, Zest AI, an AI-powered lending platform, enables financial institutions to quickly adopt AI without investing in building models from scratch. The plug-and-play nature of Zest AI's platform allows firms to tap into AI for loan underwriting and credit risk assessments, significantly shortening the time-to-market
The strategic decision to build or buy AI solutions often hinges on time-to-market and the ability to maintain competitive differentiation. Building proprietary AI systems allows companies to tailor solutions that align with specific business needs.
For instance, JPMorgan Chase has built an internal AI solution, COiN (Contract Intelligence), which analyzes commercial contracts to identify risks. By developing this in-house, JPMorgan Chase ensures that the AI system integrates seamlessly with its existing risk management processes and gives them a long-term competitive advantage.
On the flip side, companies like Goldman Sachs have chosen a hybrid approach. While building proprietary AI for their core investment functions, they rely on third-party AI vendors for tasks like HR and customer support. This mix of buying and building helps Goldman Sachs accelerate AI implementation in non-core areas while focusing its internal resources on high-impact AI innovations.
For smaller enterprises or those looking for faster AI integration, buying pre-built AI solutions offers a quick and cost-effective alternative. For example, Zest AI, an AI-powered lending platform, enables financial institutions to quickly adopt AI without investing in building models from scratch. The plug-and-play nature of Zest AI's platform allows firms to tap into AI for loan underwriting and credit risk assessments, significantly shortening the time-to-market
Data Sovereignity, Security, and Compliance Concerns
Data Sovereignity, Security, and Compliance Concerns
Data Sovereignity, Security, and Compliance Concerns
One of the most pressing concerns for financial institutions and enterprises is data security and sovereignty. Building an AI system in-house provides full control over how sensitive data is handled, minimizing exposure to third-party risks. Deutsche Bank, for example, developed its own AI systems to enhance trading algorithms while adhering to the strict data protection regulations required in the European financial sector. The ability to control and audit their AI system’s data management ensures compliance with GDPR and other global data protection laws.
Conversely, many organizations that buy AI solutions must grapple with vendor management and ensure that external platforms comply with regulatory standards.
Another example comes from the healthcare sector, where Cerner (a leading healthcare technology provider) worked with AI vendors to implement advanced predictive analytics while ensuring that patient data remains HIPAA-compliant. This illustrates how buying AI can work in highly regulated industries, provided that robust vendor management strategies are in place
One of the most pressing concerns for financial institutions and enterprises is data security and sovereignty. Building an AI system in-house provides full control over how sensitive data is handled, minimizing exposure to third-party risks. Deutsche Bank, for example, developed its own AI systems to enhance trading algorithms while adhering to the strict data protection regulations required in the European financial sector. The ability to control and audit their AI system’s data management ensures compliance with GDPR and other global data protection laws.
Conversely, many organizations that buy AI solutions must grapple with vendor management and ensure that external platforms comply with regulatory standards.
Another example comes from the healthcare sector, where Cerner (a leading healthcare technology provider) worked with AI vendors to implement advanced predictive analytics while ensuring that patient data remains HIPAA-compliant. This illustrates how buying AI can work in highly regulated industries, provided that robust vendor management strategies are in place
One of the most pressing concerns for financial institutions and enterprises is data security and sovereignty. Building an AI system in-house provides full control over how sensitive data is handled, minimizing exposure to third-party risks. Deutsche Bank, for example, developed its own AI systems to enhance trading algorithms while adhering to the strict data protection regulations required in the European financial sector. The ability to control and audit their AI system’s data management ensures compliance with GDPR and other global data protection laws.
Conversely, many organizations that buy AI solutions must grapple with vendor management and ensure that external platforms comply with regulatory standards.
Another example comes from the healthcare sector, where Cerner (a leading healthcare technology provider) worked with AI vendors to implement advanced predictive analytics while ensuring that patient data remains HIPAA-compliant. This illustrates how buying AI can work in highly regulated industries, provided that robust vendor management strategies are in place
Building an AI solution in-house can significantly contribute to long-term competitive differentiation by enabling organizations to align their AI innovations with strategic business objectives. American Express built its own AI systems for fraud detection and cardmember services. By developing these solutions internally, American Express could tailor the AI models specifically to its transaction data, giving them a unique edge in detecting fraudulent activities before they occur.
However, buying AI from external vendors doesn’t mean losing the innovation race. Companies that rapidly deploy AI solutions through external partnerships can still achieve significant competitive advantages. For example, Microsoft Azure partnered with Finastra to bring cloud-based AI solutions to financial institutions globally. By leveraging Microsoft's AI expertise, Finastra could scale faster and bring innovation to its clients without building an entire AI infrastructure internally.
A hybrid approach can offer the best of both worlds. Ping An, a leading Chinese financial services provider, has successfully blended in-house AI systems with third-party tools to scale its AI operations across healthcare, banking, and insurance sectors. This strategy allowed Ping An to maintain a competitive advantage in core AI areas while leveraging vendor expertise for non-core functionalities
Building an AI solution in-house can significantly contribute to long-term competitive differentiation by enabling organizations to align their AI innovations with strategic business objectives. American Express built its own AI systems for fraud detection and cardmember services. By developing these solutions internally, American Express could tailor the AI models specifically to its transaction data, giving them a unique edge in detecting fraudulent activities before they occur.
However, buying AI from external vendors doesn’t mean losing the innovation race. Companies that rapidly deploy AI solutions through external partnerships can still achieve significant competitive advantages. For example, Microsoft Azure partnered with Finastra to bring cloud-based AI solutions to financial institutions globally. By leveraging Microsoft's AI expertise, Finastra could scale faster and bring innovation to its clients without building an entire AI infrastructure internally.
A hybrid approach can offer the best of both worlds. Ping An, a leading Chinese financial services provider, has successfully blended in-house AI systems with third-party tools to scale its AI operations across healthcare, banking, and insurance sectors. This strategy allowed Ping An to maintain a competitive advantage in core AI areas while leveraging vendor expertise for non-core functionalities
Building an AI solution in-house can significantly contribute to long-term competitive differentiation by enabling organizations to align their AI innovations with strategic business objectives. American Express built its own AI systems for fraud detection and cardmember services. By developing these solutions internally, American Express could tailor the AI models specifically to its transaction data, giving them a unique edge in detecting fraudulent activities before they occur.
However, buying AI from external vendors doesn’t mean losing the innovation race. Companies that rapidly deploy AI solutions through external partnerships can still achieve significant competitive advantages. For example, Microsoft Azure partnered with Finastra to bring cloud-based AI solutions to financial institutions globally. By leveraging Microsoft's AI expertise, Finastra could scale faster and bring innovation to its clients without building an entire AI infrastructure internally.
A hybrid approach can offer the best of both worlds. Ping An, a leading Chinese financial services provider, has successfully blended in-house AI systems with third-party tools to scale its AI operations across healthcare, banking, and insurance sectors. This strategy allowed Ping An to maintain a competitive advantage in core AI areas while leveraging vendor expertise for non-core functionalities
Risk assessment is a critical factor when deciding between building and buying AI solutions. Uber initially relied on third-party AI vendors to optimize its route algorithms and driver-partner matching systems. However, as Uber scaled, it faced challenges related to vendor dependency and lack of customization, prompting the company to build its own AI infrastructure. This shift allowed Uber to address scalability issues and develop tailored AI models that better fit their operational needs.
Another example is Netflix, which originally used third-party AI to optimize its recommendation algorithms but eventually invested in building its own AI systems to improve user experience and scale effectively. The proprietary AI models now analyze user preferences in real-time, ensuring that Netflix can remain agile and competitive in a rapidly evolving industry.
On the other hand, smaller financial firms often lack the resources to build AI from scratch and therefore rely heavily on pre-built solutions. The decision to build or buy AI solutions in finance and enterprise is complex, involving regulatory compliance, data security, and long-term scalability. Companies like JPMorgan Chase and HSBC demonstrate the value of building proprietary AI systems for critical functions, while firms like Capital One and Bank of America show the advantages of buying AI for faster deployment and risk management.
In many cases, a hybrid approach—combining internal development with external vendor solutions—may offer the best balance, allowing organizations to scale AI effectively while maintaining flexibility and control.
The right strategy depends on a company’s goals, resources, and the role AI will play in its future growth. Whether building or buying, enterprises must stay agile, prioritize compliance, and invest in the long-term sustainability of their AI systems.
Risk assessment is a critical factor when deciding between building and buying AI solutions. Uber initially relied on third-party AI vendors to optimize its route algorithms and driver-partner matching systems. However, as Uber scaled, it faced challenges related to vendor dependency and lack of customization, prompting the company to build its own AI infrastructure. This shift allowed Uber to address scalability issues and develop tailored AI models that better fit their operational needs.
Another example is Netflix, which originally used third-party AI to optimize its recommendation algorithms but eventually invested in building its own AI systems to improve user experience and scale effectively. The proprietary AI models now analyze user preferences in real-time, ensuring that Netflix can remain agile and competitive in a rapidly evolving industry.
On the other hand, smaller financial firms often lack the resources to build AI from scratch and therefore rely heavily on pre-built solutions. The decision to build or buy AI solutions in finance and enterprise is complex, involving regulatory compliance, data security, and long-term scalability. Companies like JPMorgan Chase and HSBC demonstrate the value of building proprietary AI systems for critical functions, while firms like Capital One and Bank of America show the advantages of buying AI for faster deployment and risk management.
In many cases, a hybrid approach—combining internal development with external vendor solutions—may offer the best balance, allowing organizations to scale AI effectively while maintaining flexibility and control.
The right strategy depends on a company’s goals, resources, and the role AI will play in its future growth. Whether building or buying, enterprises must stay agile, prioritize compliance, and invest in the long-term sustainability of their AI systems.
Risk assessment is a critical factor when deciding between building and buying AI solutions. Uber initially relied on third-party AI vendors to optimize its route algorithms and driver-partner matching systems. However, as Uber scaled, it faced challenges related to vendor dependency and lack of customization, prompting the company to build its own AI infrastructure. This shift allowed Uber to address scalability issues and develop tailored AI models that better fit their operational needs.
Another example is Netflix, which originally used third-party AI to optimize its recommendation algorithms but eventually invested in building its own AI systems to improve user experience and scale effectively. The proprietary AI models now analyze user preferences in real-time, ensuring that Netflix can remain agile and competitive in a rapidly evolving industry.
On the other hand, smaller financial firms often lack the resources to build AI from scratch and therefore rely heavily on pre-built solutions. The decision to build or buy AI solutions in finance and enterprise is complex, involving regulatory compliance, data security, and long-term scalability. Companies like JPMorgan Chase and HSBC demonstrate the value of building proprietary AI systems for critical functions, while firms like Capital One and Bank of America show the advantages of buying AI for faster deployment and risk management.
In many cases, a hybrid approach—combining internal development with external vendor solutions—may offer the best balance, allowing organizations to scale AI effectively while maintaining flexibility and control.
The right strategy depends on a company’s goals, resources, and the role AI will play in its future growth. Whether building or buying, enterprises must stay agile, prioritize compliance, and invest in the long-term sustainability of their AI systems.
Join us in Hong Kong or online for our full event on 24-25 Oct 2024. Don’t miss our Digital Finance panels on 24 Oct, featuring topics as: “Navigating the Future of AI Driven Financial Ecosystems”. Hear from a world-class panel of experts on how traditional financial institutions and decentralized finance platforms are collaborating to build a unified financial ecosystem in 2025 and beyond.
Join us in Hong Kong or online on October 24-25, 2024:
Secure Your Spot: Register now to attend and gain insights from industry leaders.
Explore Sponsorship: Elevate your brand by becoming a sponsor and showcasing your leadership in financial innovation.
Partner with Us: Partner with us as a supporting organization to influence industry evolution.
Influence the Future
Prepare to contribute your insights and showcase your expertise. Our upcoming feature will enable you to shape global discussions and demonstrate thought leadership.
Sign Up for GDVC Alerts and Exclusive Insights.
*By providing your email address, you agree with our Privacy Policy.
Insights & Resources
Copyright © 2024 Global Digital Visionaries Council. All Rights Reserved.
28 Stanley Street, Central, Hong Kong
Sign Up for GDVC Alerts and Exclusive Insights.
*By providing your email address, you agree with our Privacy Policy.
Insights & Resources
Copyright © 2024 Global Digital Visionaries Council. All Rights Reserved.
28 Stanley Street, Central, Hong Kong
Sign Up for GDVC Alerts and Exclusive Insights.
*By providing your email address, you agree with our Privacy Policy.
Insights
& Resources
Copyright © 2024 Global Digital Visionaries Council. All Rights Reserved.
28 Stanley Street, Central, Hong Kong